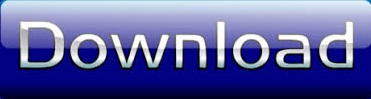
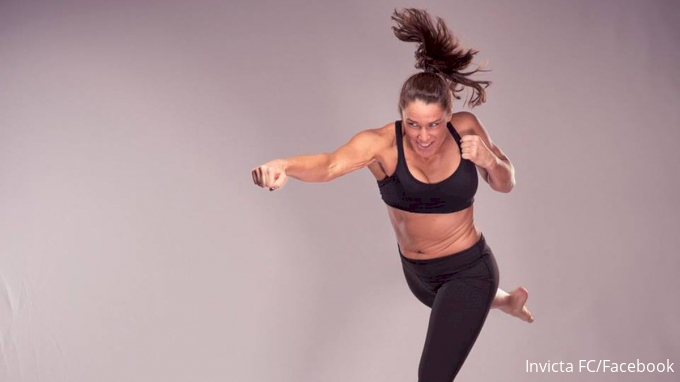
The matrix multiply inputs A and B are FP16 matrices, while the accumulation matrices C and D may be FP16 or FP32 matrices.įigure 1: Tensor Core 4x4x4 matrix multiply and accumulate.Įach Tensor Core performs 64 floating point FMA mixed-precision operations per clock (FP16 input multiply with full-precision product and FP32 accumulate, as Figure 2 shows) and 8 Tensor Cores in an SM perform a total of 1024 floating point operations per clock. Clock gating is used extensively to maximize power savings.Įach Tensor Core provides a 4x4x4 matrix processing array which performs the operation D = A * B + C, where A, B, C and D are 4×4 matrices as Figure 1 shows. The Tesla V100 GPU contains 640 Tensor Cores: 8 per SM. Tensor Cores and their associated data paths are custom-crafted to dramatically increase floating-point compute throughput at only modest area and power costs. Tesla V100’s Tensor Cores are programmable matrix-multiply-and-accumulate units that can deliver up to 125 Tensor TFLOPS for training and inference applications.
#Wmma 3 rules how to
In this blog post we show you how you to use Tensor Cores in your own application using CUDA Libraries as well as how to program them directly in CUDA C++ device code. For more information about enabling Tensor Cores when using these frameworks, check out the Mixed-Precision Training Guide. For Deep Learning inference the recent TensorRT 3 release also supports Tensor Cores. Tensor Cores are already supported for Deep Learning training either in a main release or via pull requests in many Deep Learning frameworks (including Tensorflow, PyTorch, MXNet, and Caffe2). Tensor Cores enable AI programmers to use mixed-precision to achieve higher throughput without sacrificing accuracy. Tensor cores are programmable using NVIDIA libraries and directly in CUDA C++ code.Ī defining feature of the new Volta GPU Architecture is its Tensor Cores, which give the Tesla V100 accelerator a peak throughput 12 times the 32-bit floating point throughput of the previous-generation Tesla P100. Also good are and wombatsports.Tensor cores provide a huge boost to convolutions and matrix operations. *fight descriptions courtesy of Robert Sargent of, one of the better sites for WMMA coverage. Winner: Megan Anderson by TKO (Punches & Knees) at 2:33 of round three. She backed Leibrock up with a one-two and a head kick. Leibrock countered with a triangle choke, but Anderson escaped and stood up.
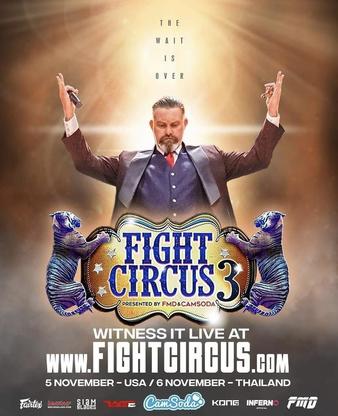
10-9 Anderson.Īnderson reversed a takedown and wound up on top in Leibrock’s guard early in the final round. She rocked Leibrock with a hard flurry late in the one-sided round. She tried unsuccessfully for a takedown and Anderson countered with a high knee. Anderson landed knees and standing elbows as Leibrock bled heavily from the nose. She mixed things up with punches and body kicks that kept Leibrock on the defensive. 10-9 Leibrock.Īnderson pinned Leibrock against the cage wall again in round two. Leibrock fought off a takedown attempt and looked for a standing guillotine choke before the bell. Anderson landed knees and held Leibrock against the fence again. Leibrock connected with more hard punches and the fighters clinched. Leibrock rocked Anderson with a right-left combo, but Anderson recovered and she landed a big flurry while keeping Leibrock pinned against the cage. Leibrock landed two right hands early on and the fighters traded body kicks.
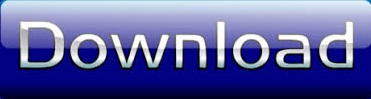